11.2. Tilburg area development case#
Python code for the Tilburg area development case (Chapter 9.1). Python code for the shopping mall design problem revisited non-linear example (Chapter 7.2).
Note
Press the rocket symbol on the top right of the page to make the page interactive and play with the code!
Import Packages#
This script is fairly similar to the non-linear shopping mall example. Only the preference functions for objective 1 and 2 are changed, together with the weights.
Note that the non-linear preference curves are created by using an interpolation function called pchip_interpolation.
import micropip
await micropip.install("urllib3 ")
await micropip.install("requests")
import sys
import matplotlib.pyplot as plt
import numpy as np
from scipy.interpolate import pchip_interpolate
from genetic_algorithm_pfm import GeneticAlgorithm
Set Weights for Different Objectives#
Set weights for the different objectives.
w1 = 1/6
w2 = 1/6
w3 = 1/6
w4 = 1/6
w5 = 1/6
w6 = 1/6
def objective_p1(h1, h2, h3, o, c, s):
"""
Objective to maximize the profit preference.
:param x1: 1st design variable
:param x2: 2nd design variable
:param x3: 3rd design variable
:param x4: 4th design variable
:param x5: 5th design variable
:param x6: 6th design variable
"""
return pchip_interpolate([80, 130, 180], [0, 30, 100], (h1))
def objective_p2(h1, h2, h3, o, c, s):
"""
Objective to maximize the amount of affordable houses preference.
:param x1: 1st design variable
:param x2: 2nd design variable
:param x3: 3rd design variable
:param x4: 4th design variable
:param x5: 5th design variable
:param x6: 6th design variable
"""
return pchip_interpolate([60, 120, 140], [0, 30, 100], (h2))
def objective_p3(h1, h2, h3, o, c, s):
"""
Objective to maximize the profit preference.
:param x1: 1st design variable
:param x2: 2nd design variable
:param x3: 3rd design variable
:param x4: 4th design variable
:param x5: 5th design variable
:param x6: 6th design variable
"""
return pchip_interpolate([60, 70, 80], [0, 50, 100], (h3))
def objective_p4(h1, h2, h3, o, c, s):
"""
Objective to maximize the amount of affordable houses preference.
:param x1: 1st design variable
:param x2: 2nd design variable
:param x3: 3rd design variable
:param x4: 4th design variable
:param x5: 5th design variable
:param x6: 6th design variable
"""
return pchip_interpolate([28, 29, 40], [100, 10, 0], (o))
def objective_p5(h1, h2, h3, o, c, s):
"""
Objective to maximize the profit preference.
:param x1: 1st design variable
:param x2: 2nd design variable
:param x3: 3rd design variable
:param x4: 4th design variable
:param x5: 5th design variable
:param x6: 6th design variable
"""
return pchip_interpolate([30, 68, 80], [100, 30, 0], (c))
def objective_p6(h1, h2, h3, o, c, s):
"""
Objective to maximize the amount of affordable houses preference.
:param x1: 1st design variable
:param x2: 2nd design variable
:param x3: 3rd design variable
:param x4: 4th design variable
:param x5: 5th design variable
:param x6: 6th design variable
"""
return pchip_interpolate([30, 35, 45], [0, 70, 100], (s))
def objective(variables):
"""
Objective function that is fed to the GA. Calles the separate preference functions that are declared above.
:param variables: array with design variable values per member of the population. Can be split by using array
slicing
:return: 1D-array with aggregated preference scores for the members of the population.
"""
# extract 1D design variable arrays from full 'variables' array
h1 = variables[:, 0]
h2 = variables[:, 1]
h3 = variables[:, 2]
o = variables[:, 3]
c = variables[:, 4]
s = variables[:, 5]
# calculate the preference scores
p_1 = objective_p1(h1, h2, h3, o, c, s)
p_2 = objective_p2(h1, h2, h3, o, c, s)
p_3 = objective_p3(h1, h2, h3, o, c, s)
p_4 = objective_p4(h1, h2, h3, o, c, s)
p_5 = objective_p5(h1, h2, h3, o, c, s)
p_6 = objective_p6(h1, h2, h3, o, c, s)
# aggregate preference scores and return this to the GA
return [w1, w2, w3, w4, w5, w6], [p_1, p_2, p_3, p_4, p_5, p_6]
Define Constraints and Bounds#
Before we can run the optimization, we finally need to define the constraints and bounds.
def constraint_1(variables):
"""Constraint that checks if the sum of the areas x1 and x2 is not higher than 10,000 m2.
:param variables: ndarray of n-by-m, with n the population size of the GA and m the number of variables.
:return: list with scores of the constraint
"""
h1 = variables[:, 0]
h2 = variables[:, 1]
h3 = variables[:, 2]
o = variables[:, 3] * 1000
c = variables[:, 4] * 1000
s = variables[:, 5] / 100
return .1 * (100 * h1) + .17 * (80 * h2) + .2 * (120 * h3) + .1 * o + .25 * (40 * h1 + 35 * h2 + 28 * h3) + .33 * c + 100500 * s + 40 * h1 + 40 * h2 + 28 * h3 + .3 * (o + (40 * h1 + 35 * h2 + 28 * h3) + c ) - 100500 # < 0
def constraint_2(variables):
"""Constraint that checks if the sum of the areas x1 and x2 is not lower than 3,000 m2.
:param variables: ndarray of n-by-m, with n the population size of the GA and m the number of variables.
:return: list with scores of the constraint
"""
h1 = variables[:, 0]
h2 = variables[:, 1]
h3 = variables[:, 2]
o = variables[:, 3] * 1000
c = variables[:, 4] * 1000
s = variables[:, 5] / 100
return 85 * h1 + 85 * h2 + 75 * h3 - 100500 * s # < 0
# define list with constraints
cons = [['ineq', constraint_1]]
# set bounds for all variables
b1 = [80, 180] # x1
b2 = [60, 140] # x2
b3 = [60, 80] # x3
b4 = [28, 40] # x4
b5 = [30, 80] # x5
b6 = [30, 45] # x6
bounds = [b1, b2, b3, b4, b5, b6]
Graphical Output#
Setup the graphical output.
# create arrays for plotting continuous preference curves
c1 = np.linspace(80, 180)
c2 = np.linspace(60, 140)
c3 = np.linspace(60, 80)
c4 = np.linspace(28, 40)
c5 = np.linspace(30, 80)
c6 = np.linspace(30, 45)
# calculate the preference functions
p1 = pchip_interpolate([80, 155, 180], [0, 30, 100], (c1))
p2 = pchip_interpolate([60, 120, 140], [0, 30, 100], (c2))
p3 = pchip_interpolate([60, 70, 80], [0, 50, 100], (c3))
p4 = pchip_interpolate([28, 29, 40], [100, 10, 0], (c4))
p5 = pchip_interpolate([30, 68, 80], [0, 30, 100], (c5))
p6 = pchip_interpolate([30, 35, 45], [0, 70, 100], (c6))
# create figure that plots all preference curves and the preference scores of the returned results of the GA
fig = plt.figure(figsize=((10,15)))
font1 = {'size':20}
font2 = {'size':15}
plt.rcParams['font.size'] = '12'
plt.rcParams['savefig.dpi'] = 300
ax1 = fig.add_subplot(3, 2, 1)
ax1.plot(c1, p1, label='Preference curve', color='black')
ax1.set_xlim((80, 180))
ax1.set_ylim((0, 102))
ax1.set_title('Municipality')
ax1.set_xlabel('Number of student houses [-]')
ax1.set_ylabel('Preference score')
ax1.grid()
ax1.legend()
ax1.grid(linestyle = '--')
ax2 = fig.add_subplot(3, 2, 2)
ax2.plot(c2, p2, label='Preference curve', color='black')
ax2.set_xlim((60, 140))
ax2.set_ylim((0, 102))
ax2.set_title('Municipality')
ax2.set_xlabel('Number of starter houses [-]')
ax2.set_ylabel('Preference score')
ax2.grid()
ax2.legend()
ax2.grid(linestyle = '--')
ax3 = fig.add_subplot(3, 2, 3)
ax3.plot(c3, p3, label='Preference curve', color='black')
ax3.set_xlim((60, 80))
ax3.set_ylim((0, 102))
ax3.set_title('Municipality')
ax3.set_xlabel('Number of remaining houses [-]')
ax3.set_ylabel('Preference score')
ax3.grid()
ax3.legend()
ax3.grid(linestyle = '--')
#fig = plt.figure()
ax4 = fig.add_subplot(3, 2, 4)
ax4.plot(c4, p4, label='Preference curve', color='black')
ax4.set_xlim((28, 40))
ax4.set_ylim((0, 102))
ax4.set_title('Municipality')
ax4.set_xlabel('Amount of offices [m2x1000]')
ax4.set_ylabel('Preference score')
ax4.grid()
ax4.legend()
ax4.grid(linestyle = '--')
ax5 = fig.add_subplot(3, 2, 5)
ax5.plot(c5, p5, label='Preference curve', color='black')
ax5.set_xlim((30, 80))
ax5.set_ylim((0, 102))
ax5.set_title('Municipality')
ax5.set_xlabel('Amount of cultural facilities [m2x1000]')
ax5.set_ylabel('Preference score')
ax5.grid()
ax5.legend()
ax5.grid(linestyle = '--')
#fig = plt.figure()
ax6 = fig.add_subplot(3, 2, 6)
ax6.plot(c6, p6, label='Preference curve', color='black')
ax6.set_xlim((30, 45))
ax6.set_ylim((0, 102))
ax6.set_title('Municipality')
ax6.set_xlabel('Open space ratio [-]')
ax6.set_ylabel('Preference score')
ax6.grid()
ax6.legend()
ax6.grid(linestyle = '--')
ax1.legend()
ax2.legend()
ax3.legend()
ax4.legend()
ax5.legend()
ax6.legend()
fig.tight_layout()
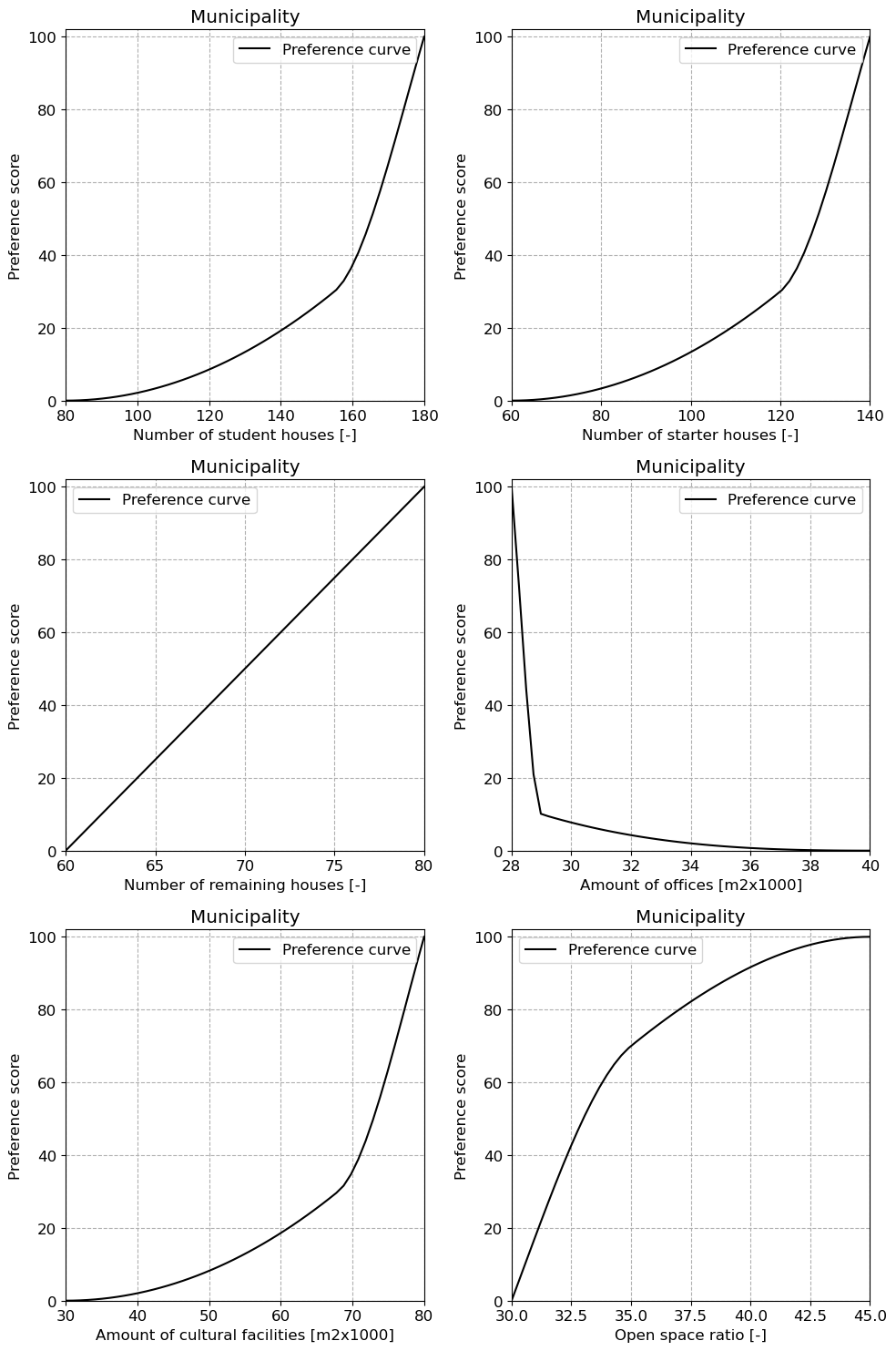
Optimization#
Now we have everything for the optimization, we can run it. For more information about the different options to configure the GA, see the docstring of GeneticAlgorithm (via help()) or chapter 4 of the reader.
Once we have the results, we can make some figures. First, the resulting design variables are plotted into the solution space. Secondly, we can plot the preference functions together with the results of the optimizations.
# We run the optimization with two paradigms
paradigm = ['minmax', 'a_fine']
marker = ['o', '*']
colours = ['orange', 'green']
# Define the figure and axes before the loop
fig = plt.figure(figsize=(12, 8))
# Creating six subplots for the six preference scores
ax1 = fig.add_subplot(2, 3, 1)
ax2 = fig.add_subplot(2, 3, 2)
ax3 = fig.add_subplot(2, 3, 3)
ax4 = fig.add_subplot(2, 3, 4)
ax5 = fig.add_subplot(2, 3, 5)
ax6 = fig.add_subplot(2, 3, 6)
for i in range(2):
# Dictionary with parameter settings for the GA run with the IMAP solver
options = {
'n_bits': 8,
'n_iter': 400,
'n_pop': 500,
'r_cross': 0.8,
'max_stall': 8,
'aggregation': paradigm[i], # minmax or a_fine
'var_type': 'real'
}
# Run the GA and print its result
print(f'Run GA with {paradigm[i]}')
ga = GeneticAlgorithm(objective=objective, constraints=cons, bounds=bounds, options=options)
score_IMAP, design_variables_IMAP, _ = ga.run()
# Print the optimal result in a readable format
print(f'Optimal result for h1 = {round(design_variables_IMAP[0], 2)} houses and '
f'h2 = {round(design_variables_IMAP[1], 2)} houses and '
f'h3 = {round(design_variables_IMAP[2], 2)} houses and '
f'o = {round(design_variables_IMAP[3], 2)} m² offices and '
f'c = {round(design_variables_IMAP[4], 2)} m² cultural facilities and '
f's = {round(design_variables_IMAP[5], 2)} open space ratio')
# Calculate individual preference scores for the results
c1_res = design_variables_IMAP[0]
p1_res = pchip_interpolate([80, 155, 180], [0, 30, 100], c1_res)
c2_res = design_variables_IMAP[1]
p2_res = pchip_interpolate([60, 120, 140], [0, 30, 100], c2_res)
c3_res = design_variables_IMAP[2]
p3_res = pchip_interpolate([60, 70, 80], [0, 50, 100], c3_res)
c4_res = design_variables_IMAP[3]
p4_res = pchip_interpolate([28, 29, 40], [100, 10, 0], c4_res)
c5_res = design_variables_IMAP[4]
p5_res = pchip_interpolate([30, 68, 80], [0, 30, 100], c5_res)
c6_res = design_variables_IMAP[5]
p6_res = pchip_interpolate([30, 35, 45], [0, 70, 100], c6_res)
# Debugging prints to check calculated values
print(f"c1_res: {c1_res}, p1_res: {p1_res}")
print(f"c2_res: {c2_res}, p2_res: {p2_res}")
print(f"c3_res: {c3_res}, p3_res: {p3_res}")
print(f"c4_res: {c4_res}, p4_res: {p4_res}")
print(f"c5_res: {c5_res}, p5_res: {p5_res}")
print(f"c6_res: {c6_res}, p6_res: {p6_res}")
# Plot the preference curves
if i == 0: # Only plot curves once
# create arrays for plotting continuous preference curves
c1 = np.linspace(80, 180)
c2 = np.linspace(60, 140)
c3 = np.linspace(60, 80)
c4 = np.linspace(28, 40)
c5 = np.linspace(30, 80)
c6 = np.linspace(30, 45)
# calculate the preference functions
p1 = pchip_interpolate([80, 155, 180], [0, 30, 100], (c1))
p2 = pchip_interpolate([60, 120, 140], [0, 30, 100], (c2))
p3 = pchip_interpolate([60, 70, 80], [0, 50, 100], (c3))
p4 = pchip_interpolate([28, 29, 40], [100, 10, 0], (c4))
p5 = pchip_interpolate([30, 68, 80], [0, 30, 100], (c5))
p6 = pchip_interpolate([30, 35, 45], [0, 70, 100], (c6))
# Plot each preference curve on the respective subplot (assuming ax1 to ax6 are defined)
ax1.plot(c1, p1, label='Preference curve', color='black')
ax1.set_xlim((80, 180))
ax1.set_ylim((0, 102))
ax1.set_title('Houses type 1')
ax1.set_xlabel('Number of houses [-]')
ax1.set_ylabel('Preference score')
ax1.grid(linestyle='--')
ax2.plot(c2, p2, label='Preference curve', color='black')
ax2.set_xlim((60, 140))
ax2.set_ylim((0, 102))
ax2.set_title('Houses type 2')
ax2.set_xlabel('Number of houses [-]')
ax2.set_ylabel('Preference score')
ax2.grid(linestyle='--')
ax3.plot(c3, p3, label='Preference curve', color='black')
ax3.set_xlim((60, 80))
ax3.set_ylim((0, 102))
ax3.set_title('Houses type 3')
ax3.set_xlabel('Number of houses [-]')
ax3.set_ylabel('Preference score')
ax3.grid(linestyle='--')
ax4.plot(c4, p4, label='Preference curve', color='black')
ax4.set_xlim((28, 40))
ax4.set_ylim((0, 102))
ax4.set_title('Office space')
ax4.set_xlabel('m2 of office space')
ax4.set_ylabel('Preference score')
ax4.grid(linestyle='--')
ax5.plot(c5, p5, label='Preference curve', color='black')
ax5.set_xlim((30, 80))
ax5.set_ylim((0, 102))
ax5.set_title('Cultural facilities')
ax5.set_xlabel('m2 of cultural facilities')
ax5.set_ylabel('Preference score')
ax5.grid(linestyle='--')
ax6.plot(c6, p6, label='Preference curve', color='black')
ax6.set_xlim((30, 45))
ax6.set_ylim((0, 102))
ax6.set_title('Open space ratio')
ax6.set_xlabel('Open space ratio [-]')
ax6.set_ylabel('Preference score')
ax6.grid(linestyle='--')
# Plot the results on the preference curve subplots
ax1.scatter(c1_res, p1_res, label='Optimal solution ' + paradigm[i], color=colours[i], marker=marker[i])
ax2.scatter(c2_res, p2_res, label='Optimal solution ' + paradigm[i], color=colours[i], marker=marker[i])
ax3.scatter(c3_res, p3_res, label='Optimal solution ' + paradigm[i], color=colours[i], marker=marker[i])
ax4.scatter(c4_res, p4_res, label='Optimal solution ' + paradigm[i], color=colours[i], marker=marker[i])
ax5.scatter(c5_res, p5_res, label='Optimal solution ' + paradigm[i], color=colours[i], marker=marker[i])
ax6.scatter(c6_res, p6_res, label='Optimal solution ' + paradigm[i], color=colours[i], marker=marker[i])
# Add legends and adjust layout after plotting all data points
ax1.legend()
ax2.legend()
ax3.legend()
ax4.legend()
ax5.legend()
ax6.legend()
# Set titles and labels for the subplots
ax1.set_title('Houses (h1)')
ax1.set_xlabel('Number of houses')
ax1.set_ylabel('Preference score')
ax2.set_title('Houses (h2)')
ax2.set_xlabel('Number of houses')
ax2.set_ylabel('Preference score')
ax3.set_title('Houses (h3)')
ax3.set_xlabel('Number of houses')
ax3.set_ylabel('Preference score')
ax4.set_title('Offices (o)')
ax4.set_xlabel('m² of offices')
ax4.set_ylabel('Preference score')
ax5.set_title('Cultural facilities (c)')
ax5.set_xlabel('m² of cultural facilities')
ax5.set_ylabel('Preference score')
ax6.set_title('Open space ratio (s)')
ax6.set_xlabel('Open space ratio')
ax6.set_ylabel('Preference score')
# Adjust the layout
fig.tight_layout()
# Display the plot
plt.show()
Run GA with minmax
The type of aggregation is set to minmax
Generation Best score Mean Max stall Diversity Number of non-feasible results
0 12.7913 734000004.3408 0 0.003 367
1 11.6111 152000012.1091 0 0.062 76
2 10.3516 78000011.2888 0 0.123 39
3 9.2537 26000010.6148 0 0.15 13
4 5.0175 18000009.723 0 0.157 9
5 3.4554 22000006.3103 0 0.16 11
6 3.1002 42000004.4105 0 0.155 21
7 3.1002 28000003.9718 1 0.16 14
8 3.0138 22000003.8417 0 0.159 11
9 3.0138 32000003.8256 1 0.157 16
10 3.0138 22000003.5345 2 0.159 11
11 3.0138 28000003.6841 3 0.16 14
12 3.0138 24000003.6805 4 0.16 12
13 3.0138 30000003.5307 5 0.155 15
14 3.0138 38000003.533 6 0.157 19
15 3.0138 30000003.5249 7 0.159 15
16 3.0138 24000003.8584 8 0.157 12
Stopped at gen 16
Execution time was 0.7476 seconds
Optimal result for h1 = 174.14 houses and h2 = 136.56 houses and h3 = 77.58 houses and o = 28.09 m² offices and c = 35.08 m² cultural facilities and s = 37.44 open space ratio
c1_res: 174.140625, p1_res: 79.43010330200194
c2_res: 136.5625, p2_res: 84.97751235961914
c3_res: 77.578125, p3_res: 87.890625
c4_res: 28.09375, p4_res: 90.29370614871809
c5_res: 35.078125, p5_res: 0.5220550998300448
c6_res: 37.44140625, p6_res: 81.91731280004475
Run GA with a_fine
The type of aggregation is set to a_fine
Generation Best score Mean Max stall Diversity Number of non-feasible results
No initial starting point for the optimization with the a-fine-aggregator is given. A random population is generated.
0 -100.0 703999988.7618 1 0.004 352
1 -86.1859 45999962.1966 1 0.062 23
2 -100.0 51999933.4575 1 0.14 26
3 -100.0 55999922.9766 1 0.147 28
4 -100.0 29999921.4447 1 0.149 15
5 -100.0 27999924.1158 1 0.153 14
6 -96.7572 43999918.2421 1 0.153 22
7 -94.9529 49999917.2364 1 0.156 25
8 -96.9928 43999913.6289 1 0.154 22
9 -100.0 45999910.4171 1 0.154 23
10 -96.8457 51999912.5267 1 0.157 26
11 -96.2197 37999911.2798 1 0.156 19
12 -96.4365 59999913.4184 2 0.156 30
13 -93.9386 75999917.3539 3 0.157 38
14 -100.0 55999908.6982 4 0.158 28
15 -94.3125 45999913.6609 5 0.158 23
16 -93.227 53999916.2058 6 0.16 27
17 -94.1088 55999915.8756 7 0.158 28
18 -93.1786 57999915.6331 8 0.157 29
Stopped at gen 18
Execution time was 1.0976 seconds
Optimal result for h1 = 179.61 houses and h2 = 139.69 houses and h3 = 79.92 houses and o = 28.0 m² offices and c = 30.0 m² cultural facilities and s = 41.19 open space ratio
c1_res: 179.609375, p1_res: 98.66712570190427
c2_res: 139.6875, p2_res: 98.6671257019043
c3_res: 79.921875, p3_res: 99.609375
c4_res: 28.0, p4_res: 100.0
c5_res: 30.0, p5_res: 0.0
c6_res: 41.19140625, p6_res: 95.041226417246
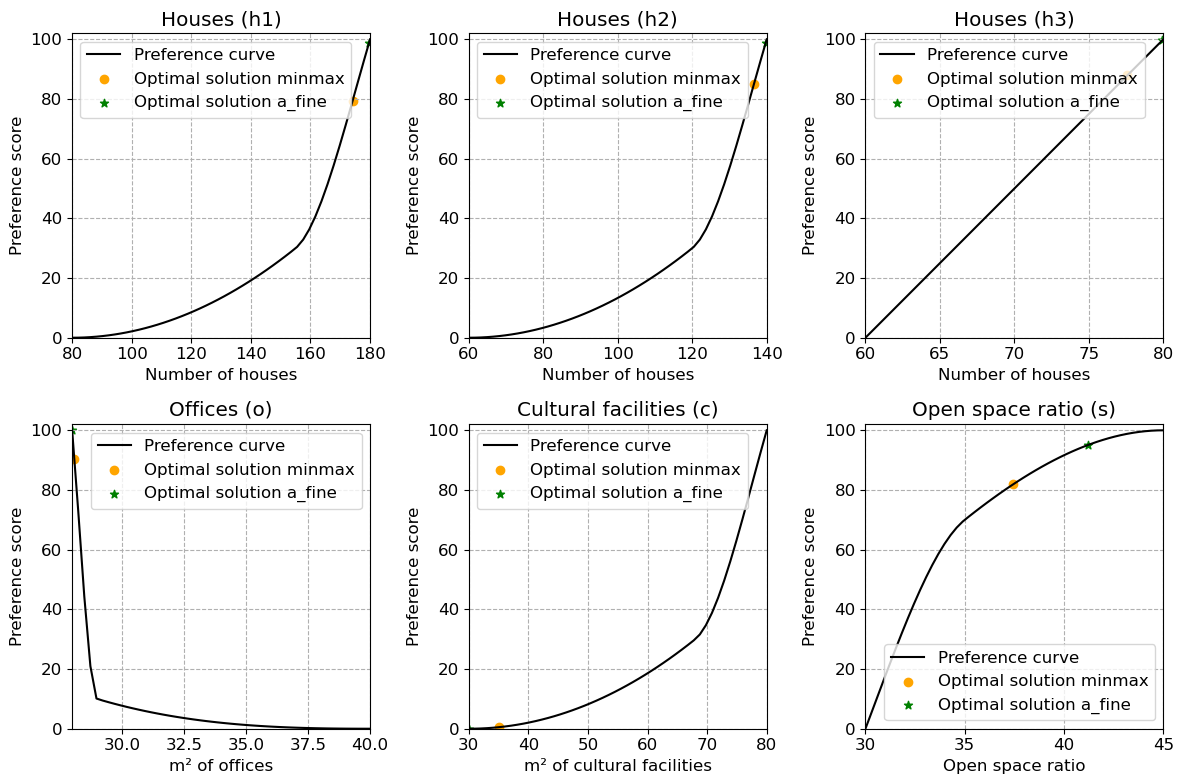